Blog Contents
hide
Introduction
Whether you love, hate or remain indifferent towards data, it’s impossible to deny its importance in today’s business landscape.
Businesses across all sectors and industries collect data and perform data analysis, to better understand their customers and business processes, in an effort to boost productivity, reduce expenditure and gain competitive advantage.
For this reason, business intelligence (BI) tools – which report on, analyze and transform these masses of organizational data into understandable and actionable information – are growing in popularity and importance.
In this blog series, we’ll explore some of the uses and benefits of data analysis when applied to a range of distinct industries and departments, and examine BI’s usefulness and potential within the retail industry.
What is retail data analytics?
The science of gathering, examining, and summarizing information about a retailer's business activities is known as retail analytics.
Retail analytics may be used for a variety of purposes, including monitoring inventory levels, assessing the success of marketing initiatives, and studying consumer behavior.
Retailers can obtain valuable insights into their customers' habits and preferences by analyzing data from various sources, including customer purchase histories, call center logs, and point-of-sale (POS) systems. This allows them to make necessary adjustments to their product offerings, pricing, return policies, and even the layout of their physical and online stores. BI software vendors that tailor their analytics solution for the retail market empower retailers to monitor these business critical information categories easily; for example, Yellowfin provides retailers embedded dashboards with fully customizable, charts and interactive elements, such as automated alerts and white-labeling capability.
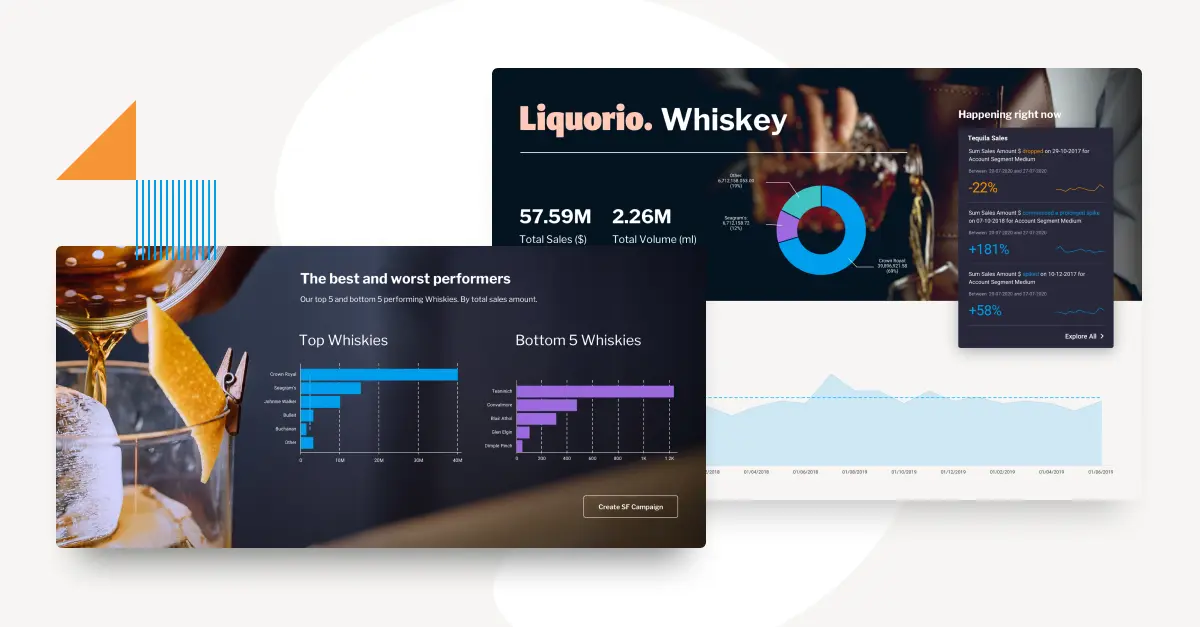
Having analytics also aids retailers in making more informed decisions regarding whether to increase and decrease staffing, as well as which marketing initiatives to prioritize and which specials to run.
In the end, data analytics benefits retailers in several important ways - by boosting revenue, cutting expenses, and enhancing client loyalty and happiness.
How is retail analytics used?
Retail analytics is another tool that retailers can use to more effectively forecast demand, diagnose problems, identify potential areas of improvement, explain past operational and financial performance, and provide recommendations, sometimes in real time, that store associates, customer service representatives, and other staff members can use to up-sell, cross-sell, or otherwise enhance their customer experience. The tools are all meant to assist merchants in increasing revenue, earnings, and customer satisfaction.
In-store analytics: Utilize data from POS systems and in-store security cameras to assist the business in analyzing consumer purchasing trends. This will allow for better arrangement of items in aisles, maintenance of proper stock levels, and lower theft rates. For instance, POS system data can reveal the success of merchandising to consumers who use their loyalty cards, while video footage can reveal whether or not customers are slowing down to look at a particular exhibit.
Customer analytics: Use information from customer-facing systems, such as chat rooms, phone logs, websites, and point-of-sale systems. Retailers may learn which products are the most popular and where they are, why certain things are being returned or exchanged, and what customer-friendly specials or recommendations work best by analyzing this data. For instance, it can assist in figuring out what promotional language works best for promoting a new product over the phone as compared to via chats.
Inventory analytics: Evaluates inventory levels for products that a shop is selling, as the name implies. It is used to recommend more effective distribution and warehousing practices, such as determining when to restock commodities based on inventory levels and anticipated demand, or if a distribution center is better to a more local storehouse. For example, inventory analytics can lower labor and transportation expenses related to carrying excess safety stock.
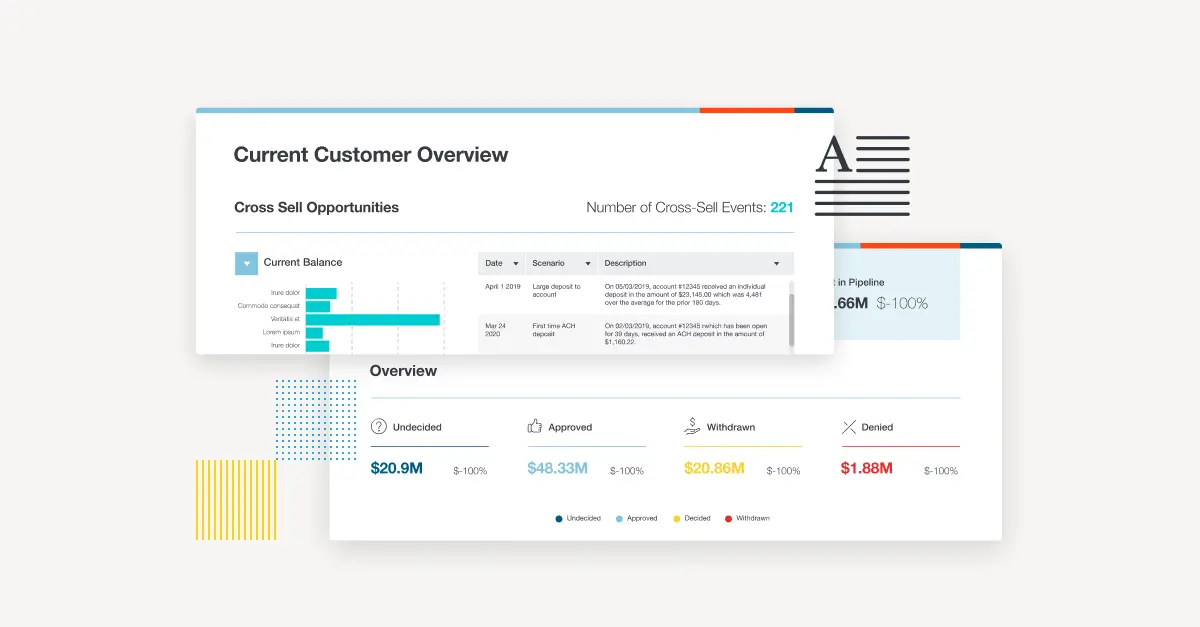
Merchandise analytics: Lets merchants assess how well they're showcasing their products, mostly in physical storefronts, in an effort to persuade customers to buy by offering attractive assortments or deals. Retailers may also modify pricing to boost profit margins across a range of items with the use of merchandise analytics.
Business intelligence (BI): Key performance indicators (KPIs) like inventory turns and sell-through rate are pre-programmed into reports, which are frequently shown as dashboards. They are mostly used to communicate top-line trends to senior management and peers.
Sales forecasting: Aids merchants in projecting future sales based on real sales data and additional variables. When combined with demand forecasting, it may estimate the aggregate demand for a product across all channels and assist merchants in making sure they have enough inventory to meet that need.
What are the types of retail analytics?
Retail data analytics may be broadly classified into four categories. Diagnostic analytics, which identifies the underlying cause of a problem, predictive analytics, which projects future outcomes, and prescriptive analytics, which suggests course corrections. Descriptive analytics aims to reflect and explain historical performance. Here is further information on each of the four methods.
Descriptive analytics: The basis for more complex analytics, such as the ones that follow in this list, is descriptive analytics. It answers the basic "how many, when, where, and what" inquiries, the kind that are often seen on dashboards and in entry-level business intelligence products that offer weekly reports on sales and inventory levels.
Diagnostic analytics: Retail businesses may discover and examine problems that might be impeding their success with the use of diagnostic analytics. Retailers may obtain a more thorough knowledge of the underlying reasons of issues they encounter by amalgamating data from several sources, including financial performance, operational measures, and consumer feedback.
Predictive analytics: Retailers may forecast future occurrences using predictive analytics based on a variety of factors, such as supply chain interruptions, weather patterns, economic trends, and new competitive challenges. This method frequently takes the kind of a what-if study, which allows a store to, for instance, determine the impact of offering a 10% vs 15% discount on a product or project the point at which it would run out of stock depending on a certain set of potential outcomes.
Prescriptive analytics: When AI and big data come together, predictive analytics results are used to suggest actions through prescriptive analytics. For example, prescriptive analytics might give customer support representatives recommendations for products they can instantly present to clients, such as an up-sell based on past purchases or a cross-sell to address a new client's request.
BI in the retail industry
As the international retail market becomes increasingly competitive, with mass offshore production and global retail conglomerates driving down prices, the ability to optimize your supply chain, react quickly to market place opportunities and satisfy customer expectations has never been more important. Therefore, accessing and maximizing the knowledge within retail data sets has never been more important.
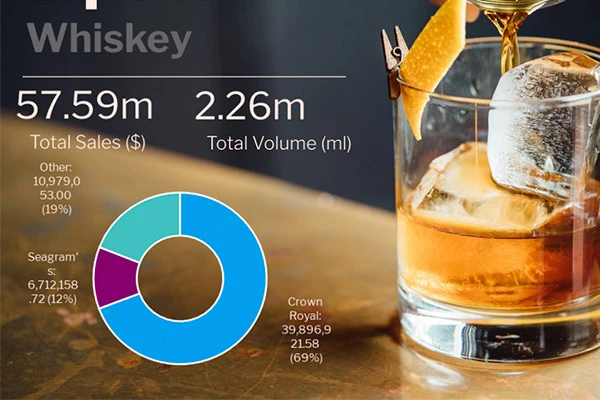
What customer-centric data should BI tools analyze and report on?
Basic customer-centric data that retailers should capture and analyze with their BI solution includes:
- Purchase types: Understand customer purchase history to help effectively promote future product offerings to customer-base
- Personal information: Help to design effective personalized marketing and communications materials/offerings using customer name, date of birth, or address, etc
- Customer feedback and sentiment: Help establish successful product types and improve future offerings
- Frequency of customer spend: Determine the frequency and type of sales and marketing promotions – distinguish impulse buyers from infrequent need-based shoppers. Also detect ‘lost’ customers and formulate strategies to ‘win’ them back
What are the key data sets that BI tools should be used to analyze and report on in the retail industry?
There are several common data sets critical to the retail industry that BI tools should be used to report on and analyze. These include:
- Sales data
- Point of sale data
- Gross margins and revenue
- Turns
- Gross margin return on inventory investment
- Market data
- Market share
- Competitor pricing
- Competitor product lines
- Competitor market share and customer profile
- Promotional and marketing data
- Success of past promotions/customer feedback
- Total cost of promotion
- ROI on promotion
- Pricing offers
- Customer-centric data
- Demographics
- Frequency
- Loyalty
- Etc (see above)
- Supply chain and operations data
- Demand for product types based on region, demographic, time of year, etc
- Identify profitable products
- Keep track of units sold (total or by category)
- Merchandising data
What are the benefits of retail analytics?
Developing accurate customer profiles and in-depth operational understandings allow retailers to predict future customer behaviors and industry trends, further enabling the ability to:
- Predict customer likelihood to purchase a new product offering
- Identify highly profitable customers by:
- Total value of sales, number of sales, estimated lifetime value
- Identify ‘lost’ customers
- Identify troublesome customers (return policies, etc)
- Identify customers responsive to promotional offerings
- Determine which customers will be more responsive to specific types of marketing
- Recognize which customers will remain loyal to your product despite changes to certain product variables (price, availability, etc)
- Increase profits
- Develop or purchase new product lines with confidence
- Develop highly targeted and successful promotional campaigns based on data collected from past campaigns and customer feedback
- Achieve accurate allocation (type and quantity) of stock across channels and stores leading to improved efficiency at the supply chain level as well as increased sales and profitability
Establishing measures
Careful data analysis of the above areas can also enable retailers to develop effective measures for:
- Assessing the components and success of marketing campaigns
- Evaluating and determining buying patterns
- Finding optimal mix of product types and quantities via region, store, season
- Deciphering optimal pricing strategies for product/category types
- Improving supply chain procedures
Moving forward
Retail operators not already using a BI application will need to consider the benefits of analytic and reporting tools to remain competitive and eliminate wastage in an industry where being in-tune with shifting customer and market trends is paramount.
How big data is transforming the retail industry
Big data is significantly transforming the retail industry in several impactful ways:
Personalization and customer experience: Retailers are using big data to understand customer preferences and behaviors, allowing them to personalize shopping experiences, recommend products, and send targeted advertisements, thus enhancing customer satisfaction and loyalty.
Supply chain optimization: Big data analytics helps retailers optimize their supply chains by predicting demand, managing inventory levels efficiently, and minimizing waste, thereby ensuring that products are available when and where they are needed.
Dynamic pricing: By analyzing large sets of data, including market demand, competitor pricing, and inventory levels, retailers can implement dynamic pricing strategies to adjust prices in real-time, maximizing profits and competitiveness.
Customer feedback and market trends: Retailers utilize big data to analyze customer feedback and social media trends, enabling them to adapt quickly to changing market demands and to improve products and services.
Fraud detection and security: Big data tools allow for the analysis of transaction patterns to detect fraudulent activities, enhancing security measures and reducing losses due to fraud.
The future of retail analytics
Retail analytics will become less apparent, less spoken about, and more commonplace in the upcoming years. Applications and users will continually utilize analytics, frequently without realizing it. This is similar to how smartphones employ location tracking all the time to swiftly satisfy user demands.
Retail analytics will become increasingly integrated into business users' everyday activities and less about creating or reviewing weekly reports. Even if they are not aware of it, more individuals will benefit from AI in their daily business dealings. Analyses of data using AI will become commonplace and not as innovative.
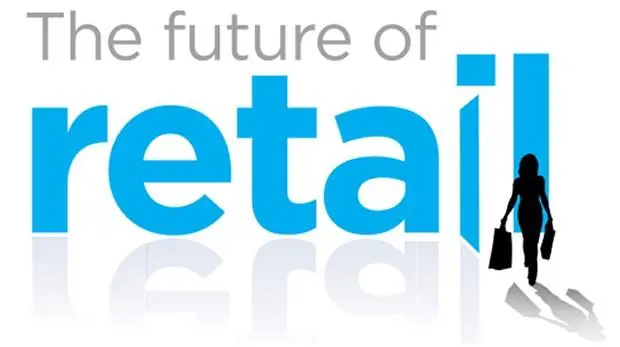
Why is retail analytics important?
One cannot exaggerate the importance of data analytics in the retail industry. It includes raising operational effectiveness, streamlining supply chains, and comprehending consumer behavior, all of which have a direct bearing on sales and client happiness. Retailers may make well-informed decisions by using business intelligence solutions to obtain insights about sales, inventory, demographics, and trends.
Big data and artificial intelligence are driving the advancement of retail analytics, which holds promise for revolutionary possibilities in terms of competitiveness and market adaptability. Adopting data analytics is crucial for success in retail.
Retail Data Analytics FAQs
How can data analytics be used in retail?
In real terms, a store may utilize data analytics to: Recognize the quantity and average value of items sold in an order. Determine which goods sell the best, the worst, and all in between. Determine who your most valuable clients are.
Which four types of retail analytics exist?
The four most popular forms of retail analytics, which are used to find new client categories and growth prospects, are diagnostic, prescriptive, predictive, and descriptive (described above).
What is the scope of analytics in retail?
Big data analytics in retail allows businesses to provide suggestions for customers based on their past purchases, which leads to more individualized shopping experiences and better customer support. These enormous data sets are also useful for predicting trends and using market analysis to inform strategic decision-making.
Which kinds of decisions does retail analytics help retailers make?
By giving business leaders advice on how much to order of a specific item, where to keep it, how much to charge for it, and what kinds of things are typically purchased together, retail analytics helps remove the guesswork out of retailing.
Getting started with retail data analytics
Learn how Yellowfin's sophisticated and user-friendly retail data analytics solutions are being used by actual users. Take advantage of our free trial to see it for yourself. Observe firsthand how people are using Yellowfin's user-friendly, retail analysis platform to get actionable insights and quickly make wise decisions.