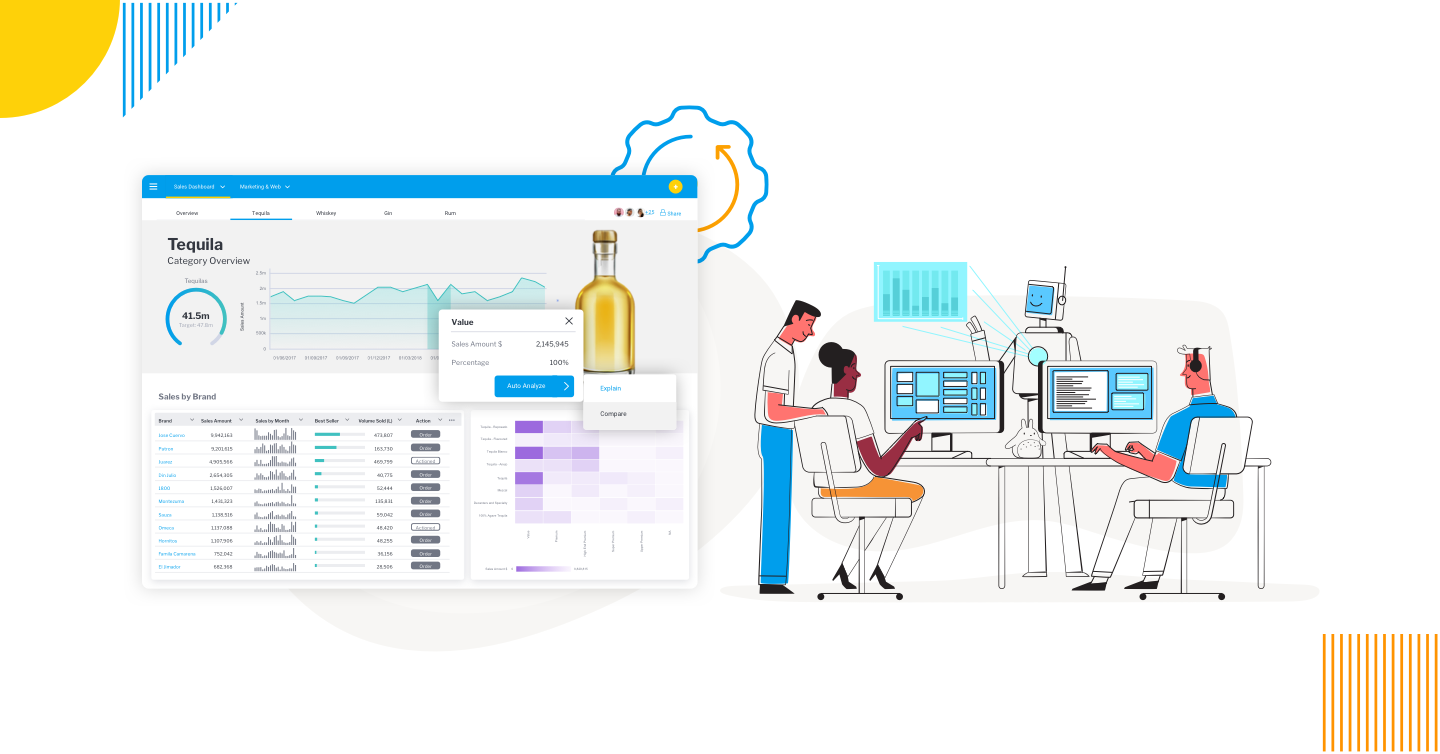
What is augmented analytics?
Analytics has become ubiquitous in our day-to-day life. It’s a key component – be that via monetization or measurement – in creating and identifying value in our business.
However, the complexity and volume of data every business accumulates is a common challenge for those that need to make decisions. Data is a continuous and always growing consideration, and it can be particularly challenging for larger enterprises.
For business users, being able to comprehensively track, identify, understand and act on what is most important – and inherently know the best action to take – is an increasingly impossible task to accomplish manually, resulting in decisions that might not be entirely data-led and rely too much on instinct. There’s an ever-pressing need to keep up with the fast-growing data we need operationally and long-term – this is where solutions like augmented analytics come in.
Today, identifying, managing and understanding data is made faster and easier through the use of automation, algorithms and natural language capabilities that enhance – not replace – the key steps along the analytics life cycle. Most importantly, it isn’t as inaccessible as you may think.
This article helps explain what augmented analytics is, how it’s becoming a key driver for many organizations ready to modernize their analytics capability – and why you should prepare today.
What does augmented analytics mean?
Augmented analytics is the use of technologies such as artificial intelligence (AI) and machine learning (ML) to transform how analytics can be built, consumed and shared.
Originally defined by global advisory firm Gartner, using augmented analytics as part of your data analytics life cycle – data preparation, data discovery, insight generation and insight explanation – is done to not only help everyone better explore, analyze, understand and act on data, but to also transform, democratize and automate the use of data for all types of users. This has created a new designation, the augmented consumer.
There’s an emphasis on ‘all’ users here, as the augmented analytics approach is designed around automating analysis processes previously typically found in specialized data science and machine learning (DSML) products. These were usually IT-led and geared toward specialists, which made augmented techniques largely inaccessible to the larger business population.
In the last few years, the proliferation of visual-based data discovery tools has seen AI and machine learning capabilities increasingly and directly incorporated within analytics and BI platforms to assist the business user specifically, rather than just data experts. This has brought together data, analytics and DSML, where they were once considered and managed separately.
For a deeper dive into the history of analytics and an explanation of its evolution into modern BI – and how we got to augmented today – we recommend watching our explainer video on Augmented Analytics Explained embedded below.
What techniques make up augmented analytics?
Augmented analytics encompasses a number of emerging, evolving and established techniques in the arena of analytics, which include, but are not limited to the following:
Augmented data preparation: These typically encompass advances in data preparation, or more typically the ETL (extract, transfer, load) phase. This can include the use of algorithms to detect schemas and joins, profiling and data enrichment through the automation of data transformation steps.
Automated analytics: Also called automated business monitoring, this typically enables the running of always-on analysis in the background. This automates the manual data discovery process and can immediately surface relevant changes in data (example – trend change), which greatly accelerates time to insight. This form of augmented analytics is particularly beneficial for large data sets with high dimensionality.
Natural language generation (NLG) and natural language processing (NLP): Natural language has become mainstream in modern BI platforms today. It automatically generates rich descriptions of insights found in your data to make complex information easier to understand and often less intimidating. These technologies may also include functionality to read and interpret text or voice, enabling the user to better engage with data through a more natural interface. Yellowfin's Guided NLQ tool aims to make asking questions of data even easier with automatically generated suggestions to build data questions, and results in natural language that everyone can understand.
Machine assisted insights: This can be in the form of machine generated visualization, calculation creation and variance analysis and is often triggered by the action of the user asking a question. For example, a user could see a spike on the chart and click on the spike to explain ‘why’ the spike occurred or compare it to another period. The machine assisted insight auto-generates the analysis, calculations and building of any charts. This process of deeper analysis may have been previously performed via filters on a dashboard (if available) or the generation of a new query to answer deeper questions.
These many capabilities are increasingly available in modern BI platforms – so much so that the Forrester Wave for Enterprise BI Platforms expects augmented analytics will dictate which providers will lead the pack heading into 2020 and beyond. According to Forrester, having a modern BI platform with the extensibility that augmented analytics offers is a critical factor that will determine the difference between an industry leader and a laggard in the long-term.
How is augmented analytics used in organizations today?
From large enterprises looking to reduce the analytic load of their teams, to surfacing risk and opportunities immediately or for software developers adding capability and value beyond traditional reporting, use cases for augmented analytics are broad.
This is particularly true for industries where the data and the variables being analyzed are complex enough or too vast in volume for their users to be able to comprehensively and reliably perform analysis with their current manual approach.
In Gartner’s report, “Top 10 Data and Analytics Technology Trends That Will Change Your Business”, the firm notes some prominent examples today.
Many in the banking sector previously targeted older customers for wealth management services, but recognized there was a gap in insights. They then used augmented analytics to automate the analysis of their high-volume data and ask deeper questions, before discovering the 20 – 35 age group were the demographic most likely to engage.
The agriculture industry reduced the time it took for data scientists to build models, using augmented analytics to find the top hybrid seed combinations to sell to farmers within a dataset of thousands, bringing time-to-insight down to days instead of months, allowing other users without technical know-how to take over the analysis process.
For the retail and eCommerce sector, Yellowfin Signals is a prime example of the extensibility of augmented analytics in modern BI. KYOWA, one of our customers in the cosmetics and health food manufacturing space, used Signals, our automated business monitoring capability, to reduce the time spent creating and managing manual reports of inventory stock by automating detection and notification of important changes relating to stock outage. KYOWA had a recurring problem with tracking out-of-stock events, as they previously manually entered and managed inventory count daily, resulting in missed items with small stock and misjudged timing to order. The data was complex to analyze due to the large number of products, so the company set up parameters within Signals to process automatic detection of out-of-stock events, taking into account specific trends with each item (e.g. the confidence interval of number of shipments).
By leveraging augmented analytics in their business, the auto-generated alerts and machine-assisted insights helped KYOWA’s users realize more efficient and precise inventory management, which resulted in prevention of any major stock-out accidents since it’s implementation. With this technology in place, stock-outs at KYOWA are currently tracking towards zero.
Top 4 benefits of augmented analytics
Having AI and machine-based analytics aren't just talking points, but a major benefit.
Augmented analytics and its forms are becoming tightly intertwined with the end-to-end analytics process, and brings numerous advantages for software vendors and enterprises. It’s vital to understand how it helps users in all stages of analysis.
Here are some of the top benefits of augmented analytics today:
#1 – Immediate automated analysis: The heavy lifting of manually sifting through huge volumes of complex data (due to lack of skills or time constraints) is significantly reduced as analysis is automated and can be set to always run. If your augmented tool finds a spike or drop or change, it can also automate the delivery of that insight, ensuring that users can then act immediately.
#2 – Faster data preparation: Augmented data preparation brings data together from multiple sources much faster. Algorithms can be used to detect schemas and joins, repetitive transformation and integrations can be fully automated, data quality and enrichment recommendations are auto-generated by the system, and you can even automate the profiling, tagging and annotation of your data before you start data preparation – and clean it for reliable analysis in a fraction of the time it takes manually.
# 3 – Improved data literacy: With natural language assisting in the explanation of discoveries and providing automated analysis of results, your users can improve their data literacy. This can assist in fostering a data-led culture that benefits the organization as a whole for the long-term.
#4 – Reduced analytical bias: Enabling the machine to perform analysis can assist in reducing analytical bias. If you don’t know what you’re looking for, you make assumptions trying to find it. Often those assumptions could lead to the use of specific data to support it. Augmented analytics can minimize potential bias often performing analysis across a wider breadth and depth of data, looking purely at factors of statistical significance. However, be aware that bias can impact the machine.
For a more detailed dive, read our blog on the practical benefits of augmented analytics.
Why is augmented analytics key for success today?
The increase in availability of augmented technology is key to understand today because it provides a far more pervasive level of adoption of analytics across the business.
Augmented analytics tools can transform the data preparation, discovery and explanation process, and open up new ways of exploring and interpretation of data.
Enabling your organization with the benefits of automated insights, utilizing machine learning and natural language for querying and exploration also doesn’t just make it easier for users or customers to consume, analyze and act on data – it gives you a competitive advantage and eliminates the risk of being left behind.
Many businesses are only now starting on their augmented analytics journey, even though this technology has been available and accessible in a number of modern BI platforms. Those who have invested in augmented have achieved significant benefit by embracing automation into their end-to-end analytics workflows. Not only have these organizations established themselves as trailblazers (when implemented successfully), they've also secured an early advantage in future proofing their analytics strategy.
Unlock Augmented Analytics with Yellowfin BI
Learn how Yellowfin BI can reduce manual analysis and provide better quality time-to-insight with our free demo - or contact the sales team for more information.