Location Intelligence: Discover how income and ethnicity affect life expectancy and poverty
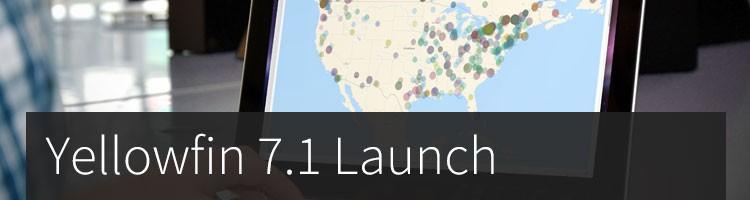
Data source:
*HealthData.gov, Centers for Disease Control and Prevention (USA), “Community Health Status Indicators (CHSI) to Combat Obesity, Heart Disease and Cancer”.
*Yellowfin US GeoPack: Contains US Census-based demographic data, which includes information such as mean household income and unemployment rates by county, as well as the necessary GIS records and geometry points to created map-based visualizations.
Data visualization:
Data visualization, Business Intelligence solution and commentary brought to you by Yellowfin 7.1: Delivering beautiful maps for brilliant insight.
It’s often said that money can’t buy happiness. But, can material wealth ‘buy’ you a longer life? Now; close your eyes, and try to conjure a stereotypical image an old, rich person. What do you see? Many of us would probably picture someone of Anglo-Saxon descent (probably male too!) – especially in a country like the USA. So does the data support this clichéd view?
Do most old, rich folk in the States in fact emulate Scrooge McDuck? Or, do such socio-economic stereotypes actually mask the truth?
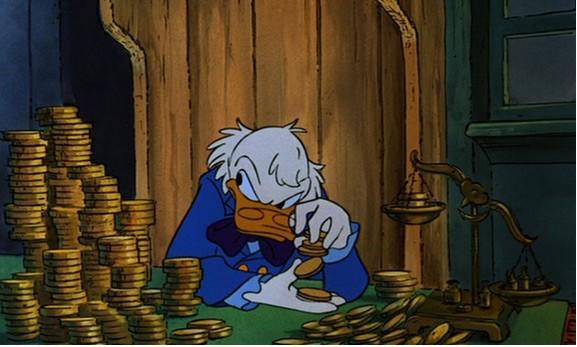
Well, to begin with, it appears that Oscar Wilde might have summed up life pretty profoundly when he notoriously remarked: “When I was young I thought that money was the most important thing in life; now that I am old, I know that it is.”
Like to see how the new ‘GeoPacks’ in the latest version of Yellowfin’s Business Intelligence (BI) platform, Yellowfin 7.1, enable you to combine your existing business data with rich location-relevant information to discover awesome map-based insights?
REGISTER NOW >
Enhance your Location Intelligence experience with detailed native base layers, zip code level analysis and rich demographic datasets.
In this instance, we’ve selected a dataset from the US’ HealthData.gov website that contains poverty rates, life expectancy and ethnicity data for every county, and combined it with our US GeoPack. The GeoPack contains US Census-based demographic data, which includes information such as mean household income and unemployment rates by county, as well as the necessary GIS records and geometry points to created map-based visualizations.
Yellowfin’s GeoPacks enable you to quickly discover rich location-based insights and correlations between existing business and spatially relevant third-party data.
Household income vs poverty rate vs average life expectancy by US county
The bottom left chart plots the mean household income, poverty rate and average life expectancy for every US county. Perhaps predictably, there is a distinct correlation between mean household income and poverty rate. For example, the vast majority of counties with a mean household income of $100,000 or more have less than 10 percent of their populace living below the poverty line.
Conversely, all counties with a mean household income of $20,000 or less have at least 11 percent of their population living below the official US government poverty line – with many suffering poverty rates above 20 and even 30 percent.
Now, here’s where it gets (tragically) interesting. It seems that Good Charlotte had it right, when they (albeit sarcastically) suggested that we should be aspiring to the lifestyles of the rich and famous.
Whilst there are a few outliers, there’s undoubtedly a clear relationship between wealth and longevity. Those counties with high mean household incomes also boast higher average life expectancies. For example, in counties where the mean household income sits at $80,000 or above, the average life expectancy never dips below 75 years. On the other end of the scale, the counties with the lowest average life spans are all clustered in the bottom right-hand-corner of the scatter plot, and are therefore distinctly associated with increased poverty rates and decreased income.
Sadly, access to quality medical care is often largely dependent on an individuals’ ability to pay for that care – a truth reflected in this dataset.
Poverty rate and average life expectancy by US county; mapped
As mentioned in our first blog in this Location Intelligence series – Location Analytics for Business Intelligence: Dude, where’s my context? – which utilized the same Centers for Disease Control dataset sourced from HealthData.gov, counties with low average incomes and low average life expectancies are also arranged in discernable geographic groupings.
For example, with the exceptions of Arizona and California State, the counties lining America’s southern border have a higher proportion of people living below the official poverty line ($23,850 total “money income before taxes” for a family of four (excluding capital gains and noncash benefits, including public housing, Medicaid or food stamps)).
Similarly, the citizens of South Dakota seem to be doing it tough, with more than 30 percent of residents in the counties of Ziebach (33%), Todd (34%) and Shannon (36%) living in poverty.
The previously identified relationship between poverty rates and life expectancy can also been seen geographically, via the average life expectancy per county map within the first post of this Location Intelligence blog series >
Straight away, we can see that counties clustered along America’s southern border, as well as those same South Dakota counties of Shannon and Todd, have lower average life expectancy compared to other regions.
While uncovering such relationships between metrics, and additional geographic groupings, is fascinating in itself, the real value is derived from the ability to ask previously unthought-of questions. So why do the counties of Shannon and Todd, in conjunction with those in the Southeast of the country, suffer from high poverty rates and low average life expectancy? Which environmental factors – be they economic, social, cultural or otherwise – contribute to these correlations and clusters?
Poverty rate vs unemployment by US county
The bottom right scatter plot on the above dashboard – within this blog – compares poverty rates, unemployment and ethnicity percentages by county.
The first thing that strikes you when looking for a correlation between poverty rate and unemployment rate is that the relationship is not as strong as you might expect. Sure, in counties where less than five percent of the populace lives below the poverty line, the highest rate of unemployment is 14 percent – with the bulk of those counties suffering unemployment levels well below 10 percent. And, at the other of spectrum, many of the counties with poverty rates of 30 – 35 percent also have high unemployment rates. But, there are also many exceptions to this rule, which make the link between these two metrics appear far from definitive.
Likewise, whilst many counties with high unemployment and poverty rates are grouped in similar locations, there are also notable exceptions. For example, while there are some very high unemployment rates amongst counties on the West and Northeast coasts, this is not reflected to the same extent when comparing the poverty rate for these same areas. Equally, a significant cluster of counties in the Southeast corner of the US – near the Mexican border – exhibit high poverty rates, but comparatively low unemployment rates. This latter point could potentially be explained by underpaid, underemployed or even illegal migrant workers who have crossed the US-Mexico border to find work.
Whilst there could also be a raft of other factors that influence the geographic dispersion of these measures, its important to realize that the absence of an expected pattern or connection can also prove just as revealing as a strong link between two or more metrics. But, without the ability to map this information, how would you discover such patterns – or lack thereof – in the first place?
Poverty rate vs unemployment rate vs mean income vs average life expectancy by ethnicity per US county
You’ll also notice, using the tool tips when moussing over either of the two maps within the above dashboard, that we’ve included the majority ethnic group (as a percentage of the total population) for each county. So what happens when we filter this dashboard by the majority ethnic group for each county?
Assessing US counties by ethnicity: Counties with a majority Hispanic population
When filtering our dashboard by counties that have a majority Hispanic population, a few patterns become immediately evident. Most of these counties are situated along the US-Mexico boarder. Additionally, the aforementioned disparity between the unemployment rate (relatively low) and poverty rate (relatively high) within many of these counties is again highlighted. This finding adds weight to our hypothesis that many of the people working in these counties are immigrants who are either underpaid, underemployed or even illegally employed. Additionally, if you’re an illegal immigrant, it’s highly unlikely that you’d report your state of unemployment to the government in an effort to receive welfare assistance.
Whilst reported poverty and unemployment rates only demonstrate a mild correlation at best, what does stand out is this: Only one county with a majority Hispanic population (San Benito, CA) has less than 10 percent of its residents living below the official poverty line.
The scatter plot on the left-hand-side of the dashboard demonstrates a straight-forward, and logically anticipated, relationship between the mean household income and poverty rates within these majority Hispanic counties.
Where to next?
Use the filters on this embedded dashboard to filter the reports by different ethnic groups, and send your insights and discoveries into pr@yellowfin.bi. We’d love to hear from you.
And, if you’d like to find out more about how demographic data and mapping can enhance the usefulness of your corporate data and data analysis, be sure to register for the launch of Yellowfin’s new BI platform, Yellowfin 7.1.
REGISTER NOW >
Attend to discover a BI platform that makes world-class Location Analytics quick and easy with unique mapping capabilities.
Locate new opportunities fast with detailed native base layers, zip code level analysis and rich demographic datasets in Yellowfin 7.1.