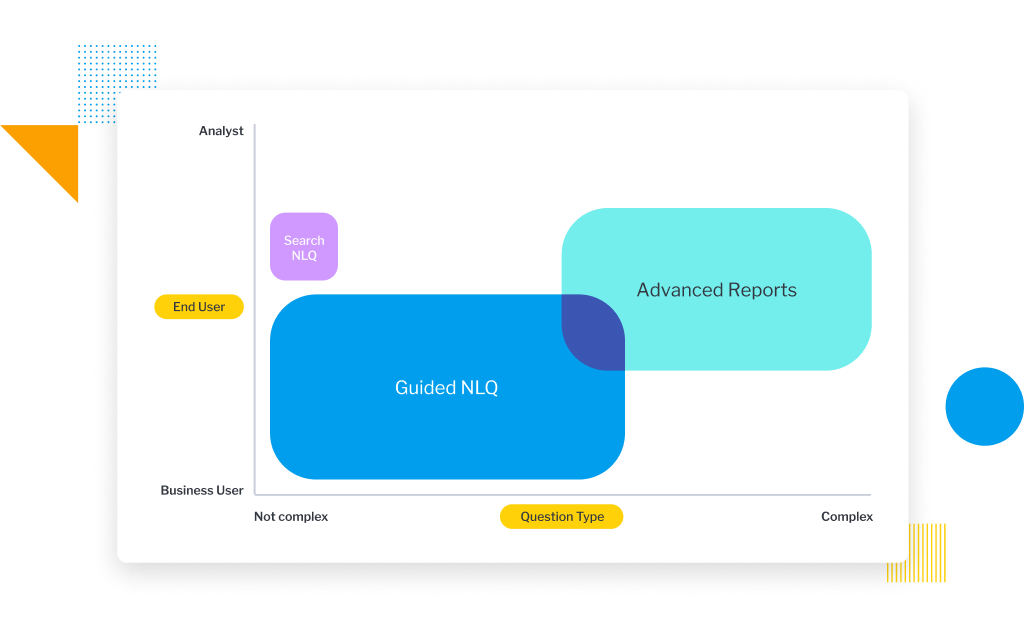
Why natural language query (NLQ) didn’t take off
Providing the ability to ask questions of data is useful, but only if you can guide analytics users toward the right answers.
Over the last couple of years, the market has been really excited about natural language query (NLQ). A lot of vendors have brought products to market, and the analyst community has also seen NLQ as potentially being able to solve a particular problem that's been in the BI space for a long time - but it hasn’t managed to achieve that just yet.
In this blog, Yellowfin CEO Glen Rabie shares his thoughts on the search-based approach natural language query, and how Yellowfin are approaching it in all-new, guided way.
The problem with natural language query today
The main problem that analytics vendors have tried to solve with natural language query is the ability for business users to ask simple questions of their data and get an immediate answer. The current solution has been to give users a search bar, similar to Google, where they can type in a question, but this doesn't solve the core problem.
A business user might want to ask a question, such as ‘what were my sales this year, compared to last year in Europe?’. That's a very simple question to ask, but very hard to do in a traditional UI environment because a textbox leaves everything open to interpretation. There is so much ambiguity in the question, and it can be difficult to work out exactly what a person means. The system can’t determine which data set they're trying to query, or what the context of their data is, or the synonyms they may be using.
Following this typical approach to NLQ means as a vendor, you spend time trying to solve the semantic problem rather than the analytical problem of their user. As a result, many vendors have limited the scope of what a user can do with the search tool and data you can access with. This means the natural language solution has limited value to the user and that’s why we haven't seen the take up and success of these types of tools.
At the other end of the spectrum, you have drop down menus or drag and drop. These are typical analytical tools where the user can interact with the UI, choose specific outcomes and build a report. This solution can make it very difficult for business users to get the answer they want because of the way the data is structured. You often have to have extensive prior knowledge, or be an expert in the tool to answer the question.
3 key challenges of search-based NLQ
Here are 3 examples of real-world challenges with the search-based approach to NLQ.
1. It's expensive: Search-based NLQ parses the keywords in a query, and matches them with elements in related databases - a concept familiar to anyone who has ever used Google or a search engine for questions. But mapping those responses to business data is hard, and high cost. The Gartner 2021 Hype Cycle for Analytics & Business Intelligence found with search-based NLQ the effort to map and model data were too high, resulting in the questions supported often too basic to be useful, or yielding inaccurate results.
2. Lack of other integrations: Analytics solutions on the market with NLQ don't typically support analytically complex queries, data sizes and types, have integration with natural language generation (NLG) to present explanations of findings in a user-friendly way, or have automated insights that can quickly show the user related findings.
3. Immature: Many NLQ offerings don’t support languages beyond English, and don’t provide examples of next questions to ask to help the user further their search journey. They also don’t know how much information needs to be predefined in advance, and how much needs to be dynamically generated, and are still working out the needed balance.
These obstacles are why Yellowfin believes a guided query experience, not a search-based one, is the best way to leverage the amazing technology of NLQ and all its possibilities, to open up analytics to all users - not just analysts or experts.
How Yellowfin is changing NLQ with guided analytics
At Yellowfin, we're taking a completely different approach to natural language - one that guides users toward the right questions to ask of their data, to get the right answers.
We're thinking about how to meld the complexity of UI and search and bring it into a new paradigm. We want to create a user experience that gives business users the freedom to explore their data, and be guided to get the answers they want quickly. Our solution will get them to data on their own without the need for an analyst to assist. Most importantly, it will be another key method for self-service users of all skill levels to gain insights.
While what we have in store is very different to what’s in the market already, we're looking forward to introducing Guided NLQ, and changing the way you discover insights.
Read: What is natural language query, anyway?
25% of enterprises will use some form of natural language technology in their business by 2024. Brush up on your knowledge of NLQ, and learn the difference between guided and search NLQ, in our extensive blog guide.