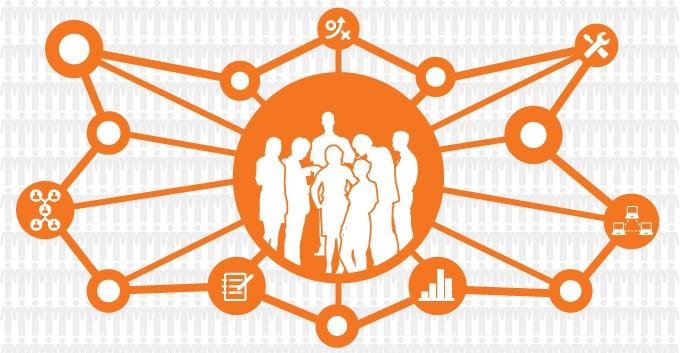
Marketing analytics: Why marketers should think multi-attributes when segmenting a customer base
By analytics professional and Yellowfin user Rohan Wickramasuriya
This post was syndicated from Rohan Wickramasuriya’s DPP Analytics blog with permission from the author. The original post can be found HERE >
Find out more about Rohan and his professional career HERE >
Customer segmentation for marketing analytics
Customer segmentation is a frequently discussed topic in marketing and marketing analytics. It primarily involves identifying groups of customers who exhibit similar behavior (e.g. purchasing) with respect to a product or service offered by an organization.
While there are numerous posts online discussing customer segmentation, not many clearly explain the need to simultaneously consider multiple attributes in this exercise. This post attempts to explain how marketers can benefit from multi-attribute segmentation.
Let’s assume your company has several products, out of which A, B and C have been recently introduced. Based on the characteristics of the customers who already own these new product types, your marketing department wants to identify other customers in the base who would be interested in the same.
What’s very common in a segmentation exercise like this is to look at how a customer attribute like age varies across products. In the best case scenario, we would be able to see products A, B and C are owned by customers of distinct age groups (Figure 1).
Figure 1: Ideal segment separation in a single dimension
If the observation was similar to Figure 1, you could have easily worked out that the customers in the age range 20 – 33 are certainly interested in product A, and so on.
Unfortunately, a single attribute is almost always insufficient to segregate the customer groups of interest. With respect to our hypothetical example, you’re more likely to observe an age distribution across products similar to the below.
Figure 2: Likely segment separation in a single dimension
According to Figure 2, customers of similar age own more than one product type, particularly products A and B. Older age groups, however, seem to prefer product C.
In a situation like this, your next step should be to look at another attribute, but not in isolation. Rather you should use a second attribute together with the first (age) to help you discern the segments.
Ideally, this second attribute should encapsulate completely different information about the customers. You may have heard analysts referring to this as ‘uncorrelated variables’. Again in practice, it’s rare to find completely uncorrelated variables. Hence, we should go for variables that are not ‘highly’ correlated. We will squash this vague term ‘high’ in another post. For now, let’s say you have the second attribute ‘income’ and you decided to do a scatter plot using age and income attributes (Figure 3).
Figure 3: Segment separation in 2-dimensional space
Now you see that the customers who own product C are fairly well separated from the other two groups. We still have some more work to do to gain a good separation between A and B. Let’s bring in another attribute that provides significantly different information about your customers, and look at the product separation in a three-dimensional space (Figure 4).
Figure 4: Segment separation in a three-dimensional space
In Figure 4, you can see segments A and B are now fairly well separated. Obviously, you could improve this further by adding few more attributes. As humans, we’re limited to visualizing three dimensions at a time. However, this is no limitation to modern computers. We can ask our computers to use significantly more than three attributes to achieve segment separation in high dimensional space.
One drawback associated with multi-attribute segmentation is that the rules that set the segments apart tend to be numerous and complex. Luckily, there are tools that can help us figure out such rules fairly easily. Below is a decision tree created to work out those if-then type rules for segmenting our hypothetical customer base.
Figure 5: Decision tree that can help segment the customer base
This rule set is actionable, because we can apply it to the whole customer base and identify other customers who are likely to be interested in products A, B and C.
The accompanying tutorial will take you through the steps required to create a decision tree similar to Figure 5. You can find the tutorial HERE >