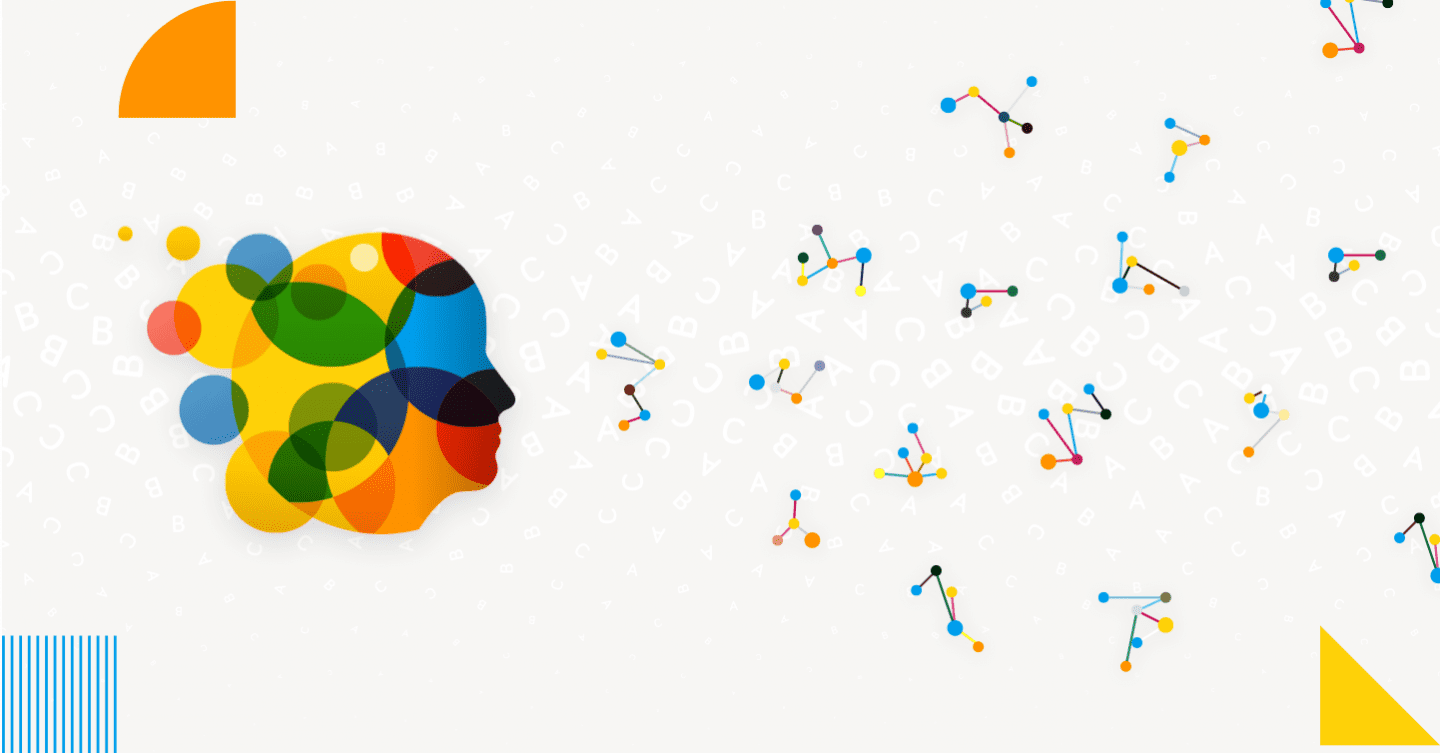
What is Natural Language Generation? How NLG Works in Analytics
From artificial intelligence (AI) to machine learning (ML) to conversational chatbots, the tools we use to interact with and consume information are rapidly changing thanks to powerful new technologies that make understanding our data more accessible than ever.
One particularly influential field is natural language technology (NLT) and its branches.
With its global market projected to grow at a CAGR of 21.8% from 2024 to 2030, many businesses will be using some form of natural language generation technology (NLG) within the next decade. But what exactly is NLG, in the context of embedded analytics solutions, and how can it be used to help enhance our users' data analysis processes?
What is the meaning of natural language generation (NLG)?
Natural language generation is best described as a sub-type of artificial intelligence that generates linguistically rich descriptions of insights, both written and spoken, in plain English. NLG does this by scanning and finding the most important concepts in structured data that resides in our databases or apps, and translating it into a consumable, text-based narrative easier for business users to access and understand.
Essentially, NLG describes a new way of users consuming insights - by reading a helpful auto-generated summary that uses easy-to-understand plain English to make the process of data analysis more accessible for more people (non-technical and experts).
Many BI and analytics platforms today are increasingly integrating natural language technology to help users when they opt to query their data for answers. Yellowfin leverages NLG to power its exclusive Assisted Insights feature, which automatically analyzes data to generate insights in digestible dot-point summaries and charts.
How does Natural Language Generation work?
Natural language generation is a branch of natural language technology (NLT), sitting alongside natural language processing (NLP), natural language understanding (NLU) and natural language query (NLQ), that is often used as part of modern analytics solutions.
These broad areas of technology encompass capabilities such as text analytics, machine translation, text summarization, language knowledge graphs, and more advanced techniques. While possible for some time now, the rise of AI and machine learning (ML) in data analytics has led to NLT being leveraged to enable greatly improved functionality of our business intelligence and analytics tools, including the way we analyze our data.
Yellowfin BI's Assisted Insights feature uses NLG to provide auto-generated analysis.
In the past, NLG generated relatively basic answers for user queries based on preset grammar and semantic rules, typically hand-in-hand with slot filling, to produce textual output. There are many traditional (and useful) examples of basic NLG that translate data into text, using template-based systems with rule-based functions that insert variables like dates, words and times into auto-generated, straightforward translations.
Today, many modern examples of natural language generation use AI programming, computational linguistics, deep neural network methods and machine learning algorithms to produce its written or spoken narratives, which results in a more conversational, expressive “human-like” tone. NLG can also now access more complex structured data sources than in the past, such as API calls, JavaScript Object Notation (JSON) feeds, and real-time feeds with users, such as chatbots.
Through these many advancements, NLG can theoretically and more reliably provide users with slightly more sophisticated answers that don’t just translate the facts within data. It can now:
- Express findings from analyzed data in a conversational, human-like manner
- Highlight interesting nuances and hidden patterns that may prove insightful
- Provide both summary and detail-level explanations each user can request instantly
Because NLG uses automation, it is also capable of mining vast volumes of data and generating insights from our data faster than a user may be able to decipher and communicate manually.
The rich language that is produced by analytics solutions with some form of NLG capability are also commonly accompanied by auto-generated data visualizations, such as charts, graphs and tables; like the textual narratives, these visualizations provide users with additional helpful context and help inform them on what is most actionable. This is what Yellowfin aims to provide with its NLG-powered Assisted Insights analysis feature.
The business use cases for NLG
Natural language generation is useful where there is a need to generate text-based narratives or spoken content from business data, which is why it's in many BI tools.
NLG is most commonly deployed as a helpful enhancement for self-service analytics. For example, business users exploring a dashboard on revenue performance can query their data and get text-based explanations and comparisons to lead them toward hidden patterns they may not have known to explore and to additional insights - theoretically.
NLG can also be used to conduct routine analysis reporting tasks that otherwise require a lot of time to do; a retail business can use it to generate product descriptions, while sales could generate high-level text analysis of monthly performance instead of writing it up manually. The effectiveness of this is dependent on the implementation of NLG.
Finally, NLG is often used to supplement virtual conversational assistants (like chatbots or voice assistants) to produce human-like conversations with customers, though its capability to produce natural responses in spoken form is less developed at this time.
The dynamically generated answers and narratives provided by NLG tools can compare or summarize important information and communicate it in a human-like manner to provide more meaningful answers to a user’s query; they also make it easier for the user to consume. Most importantly, natural language focuses on providing users with critical context to otherwise complex data points, with both high-level and detailed explanations.
This means more people, like non-technical users, can quickly understand an important report or complex chart or table without relying on analysts to explain it.
The business benefits of NLG in analytics
While still evolving and proving its value, the potential benefits of NLG tech are clear.
Decision support: Natural language generation improves the efficiency and effectiveness of the average user’s decision-making process while using their analytics tools. It supplements their existing knowledge and skillset with instantly generated summaries, detailed explanations and comparisons of the data they need answers from.
Insight interpretation: People possess different levels of analytical understanding. For the average user, having an option to generate textual narratives to their important questions through capabilities like NLG help them more reliably and accurately interpret data, and point them toward unseen patterns or hidden nuances they may not be able to find manually. If a user prefers reading compared to visual communication of data, natural language generation can open up and help them interpret complex data, such as statistical relationships, far easier.
Time savings: There are many repetitive reporting tasks, like writing summaries of business data, that take a lot of time for regular users to complete manually. Natural language generation can help increase efficiency by generating specific information quickly, without requiring a user to spend as much time manually analyzing data or seeking assistance from IT or analysts.
Why is NLG used in analytics platforms?
For many users, the text-based narratives of natural language generation can be preferable to graphical interfaces or rich visualizations when they are trying to find the answers they need. Combined with capabilities like contextual analytics, it's easier to understand the data presented on a dashboard or report if the tooling explains it for you.
The reality is not everyone thinks numerically. Some people tend to prefer reading, for example. When having to interpret a visualized chart packed with metrics, they can get frustrated or might not be able to glean the answers they need from their data.
Lawyers are good examples of readers, as they read and write a lot for their role. You wouldn’t throw them numbers, charts or heavily statistical reports to explain cases to them; this is where the generated explanations of natural language can help greatly.
Yellowfin Assisted Insights can help cater for a broad range of different BI users with its use of NLG techniques to generate both helpful visualizations and readable text-based narratives for users who might need additional high-level or in-depth detail to interpret the many metrics presented to them. The user can simply click on parts of their data to 'auto-analyze' what's presented, and the system then generates a textual explanation or comparison of insights instantly, helping to form a better interpretation.
What Does NLG Look Like in Analytics?
Discover how you can leverage the benefits of NLG with auto-generated visualizations and detailed textual explanations and comparisons with Yellowfin Assisted Insights.