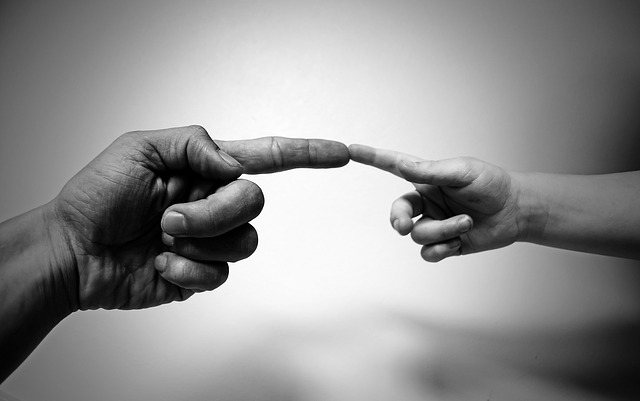
The 3 phases of automated analytics maturity
When we look at automation in the BI industry and how vendors are tackling augmented analysis, or automated analytics, there are three distinct phases of maturity:
Phase 1: Building better dashboards
The first phase of automated analytics maturity is the workbook model where users load data into a platform, and then automation helps them build a better dashboard. Often the data is loaded manually so it’s not live. If a business user doesn’t have access or knowledge of the backend, then they must rely on data analysts to keep the underlying information updated. Once the data is loaded, the platform then runs some algorithms that make layout suggestions based on the data. These tend to be simplistic models - there’s no time series based analysis or anything complex.
The person that benefits in this phase is the analyst. The platform helps them get part of the way in their analysis and gives them suggestions about how to build a better dashboard. But this phase doesn’t help the business user to discover insights. Business users are not told when something changes or if there’s a new trend in their business. The platform only looks at the data at a point in time so it isn’t continuously looking for changes like automated analytics would.
Most vendors like Tableau, Qlik and Power BI have built this automated suggestion capability for dashboard layouts.
Phase 2: Assisted insights
In the second phase of automated analytics maturity, users can ask a question and the application then analyzes the data and tries to find an answer. Fundamentally, the investigation is user-led.
This capability assumes that the user knows what questions to ask the platform - if a user has seen an outlier or a spike it may trigger them to question it. The platform doesn’t do the heavy lifting of finding outliers for the user. Instead, the platform just automates the existing data discovery process, but the onus of understanding what questions to ask still sits with the user. That’s why this capability is used mostly by data analysts rather than business users.
One of the biggest challenges in analytics is knowing where the problem is and then directing the product to look there. The platforms in this phase don’t address this issue. Often, the user ends up with too much information. Salesforce Einstein is a good example of this. It delivers pages and pages of information that are statistically relevant. But in practice these insights are completely irrelevant because they’re not actionable or their potential impact is miniscule.
Platforms that have some form of assisted insights include ThoughtSpot, Domo, IBM Watson, and Yellowfin’s Assisted Insights feature.
Phase 3: Identifying outliers and trends
In this phase, the platform automatically detects outliers and trend changes and brings these to the attention of the user. This is mature automated analytics. It’s a big challenge for vendors to develop this capability because it isn’t just about the tech. The user changes from being an analyst to a business user and that requires a completely different way of thinking - the user experience needs to be reimagined.
The tech is also more challenging because there’s a lot of clever stuff that goes under the hood when you start to think about what makes a data signal important. You have to know a lot about the dataset that you're querying to be able to explain a change. This means the platform must do a lot of analysis to surface the right information.
To be successful, the automation has to determine whether something has enough value to a user to display it to them. In a large, complex business the value can differ depending on who you show it to. Something may be insignificant to the CEO, but very important to someone further down the organization. Getting this right is challenging but it’s also where the real value of automated analytics is generated.
This phase also breaks the reliance on the dashboard. Instead of logging into a dashboard, the platform delivers signals that are hyper-relevant directly to the business user as they happen. This is the future of analytics.
We’re in the early stages of delivering this automated analytics capability through Yellowfin 8. Apart from Yellowfin, the only vendors in this space at the moment are startups like Outlier. But we’re already seeing large vendors buying out small startups to get their ideas as early as possible - Salesforce bought Datorama and BeyondCore, and Workday bought Stories. The light has clearly gone on in the industry that automated insights are the future.
Automation in BI is ultimately being driven by skill shortages in the industry. There aren’t enough data analysts to go around so everyone is trying to automate as much of the process as they can. But I believe the next phase will go much further than just automating mechanical tasks. It will mean users won’t need dashboards anymore because important insights will find them, and that’s exciting.
5 important BI trends for 2019
Here are the five key trends emerging in the analytics space over 2019.