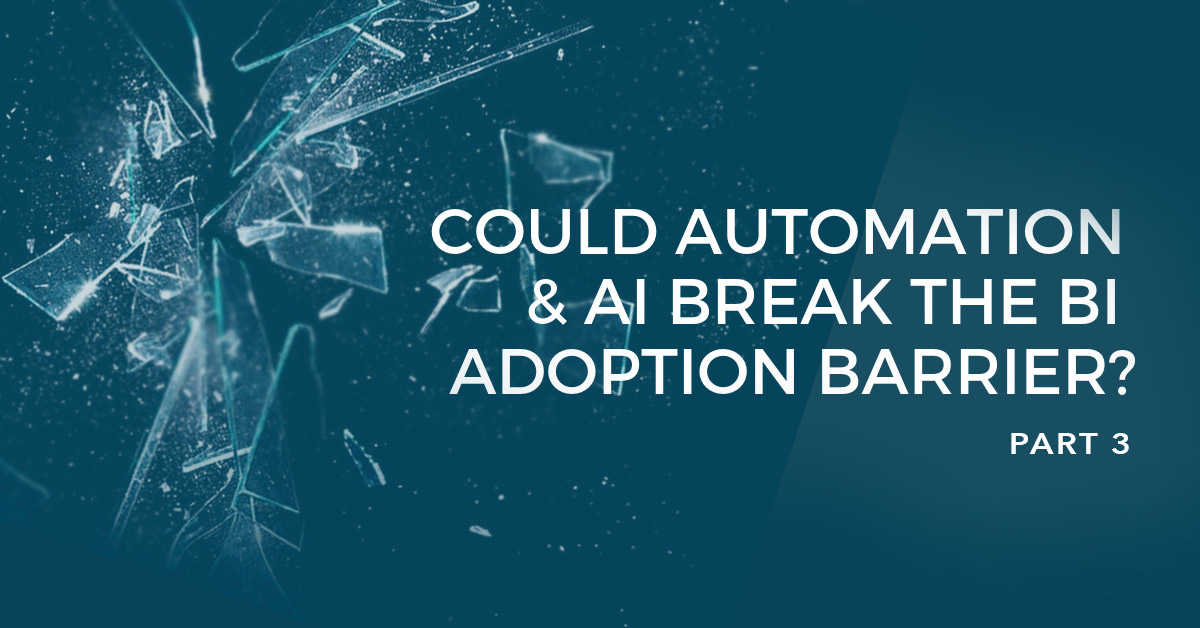
Part 3: How machine learning, AI and automation could break the BI adoption barrier
In the first blog post of the series, we saw the dire state of analytics adoption. This problem feeds into the low usage and governance of data across organizations. Then, in the second post, we saw how the evolution of analytics has brought us to a prime position for augmented analytics. But will this new wave of augmented analytics break through the barriers to BI adoption? First, we need to understand the barriers you face today to getting analytics widely adopted in your organization and therefore creating a data-driven culture.
The four main blockers to BI adoption
Here are the four fundamental issues that have been seen time and time again in enterprises and can be seen in the results of the Computing Research survey.
1. Tools are not built for the wider business and business user
However intuitive the interface of a BI tool is in comparison to past versions - and there are many excellently designed tools - there will still be a requirement for training to enable people to use it.
As Figure 3 shows, 38% of respondents said that the difficulty of using tools is a key factor in preventing wider use to drive decision making. This leaves the wider enterprise either creating all their own analytics on ungoverned spreadsheets or relying on the analytics team and a few trained power users for all their access to the data analytics and insights. People do not have the time or inclination to use the tools for themselves if they are hard to use. Yes, training is available, but time spent training is time out of their main role.
What is stopping more people from making use of analytics tools to drive decision making? (Choose all that apply)
2. A lack of people skilled in interpreting data
As the complexity and volume of analysis become more sophisticated, so does the skill required to analyze that data and unearth the action points. A lack of skills in interpreting data is the biggest barrier to adoption according to the Computing Research survey with 58% of respondents stating this reason.
"72% of global data and analytics decision makers say that they can access the data they need to obtain insights in a timely manner.
However, even the most modern BI tools that make data more accessible still require significant subject matter expertise to find the right data, ask the right question, and interpret the results correctly in order to achieve tangible business outcomes."
Forrester, 'AI Unlocks the Business Intelligence in BI'
Business users are not trained analysts and they don't always understand the full context of the data and visualizations presented to them. It is also not their job to spend hours analyzing the data and building self-service reports. The enterprise wants its salespeople selling, not doing data analysis. This means that data is either not consumed at all, or not consumed correctly because the data is not understood, or it has been created in self-made, ungoverned spreadsheets that are error-prone, creating mistrust in data.
3. The process of getting to insights takes too long
'The data team is time-constrained' is the third-most cited reason for lack of adoption in the survey at 43%.
A lot of hard graft goes into providing basic data visualizations and digging through the data to find actionable insights. The data preparation, transformation, and delivery take time - time that business users cannot afford when they need to make strategic, data-driven decisions quickly. And even after all the time is spent on getting clean, reliable data to the user, the data might not deliver insight into why an event has occurred. It is not good enough anymore to know what has happened; the business needs to know why.
Because the tools are not accessible and few people are skilled in using them, the workload lands on a comparatively small number of people. With more and varied data at a higher velocity, the load on the analytics team only continues to increase, squeezing their time further and stretching their resources.
4. The value of predictive analytics is still hard to determine
A final barrier is the issue of demonstrating the value of predictive analytics. As shown in Figure 4, businesses are prioritizing the reviewing of ML and automation tools to unlock insights, bringing data models into production, and hiring a data scientist. But it has been difficult, until very recently, to show the value of these investments.
What are your top analytics priorities?
Enterprises that employ data scientists often find that the predictive analytics produced by data scientists in data science tools is often a silo. The business has little understanding of what they do, how the data science impacts the main business, or what the value of the models is to the enterprise. Models are built, but they are often not put into production with corporate data, so they never make it into the 'real' world, which severely limits their value.
As former data scientist of Airbnb, Robert Chang, said:
"All of my work was done in my local machine in R. People appreciate my effort but they don't know how to consume my model because it was not 'productionized' and the infrastructure cannot talk to my local model. Hard lesson learned!"
Until these issues are solved, we will not see the adoption of analytics increase within enterprise businesses and decision making will continue to be missing the empirical data it needs.
Read the final part of the series: Part 4 >
< Read Part 2: The evolution of analytics
< Read Part 1: The state of analytics adoption today