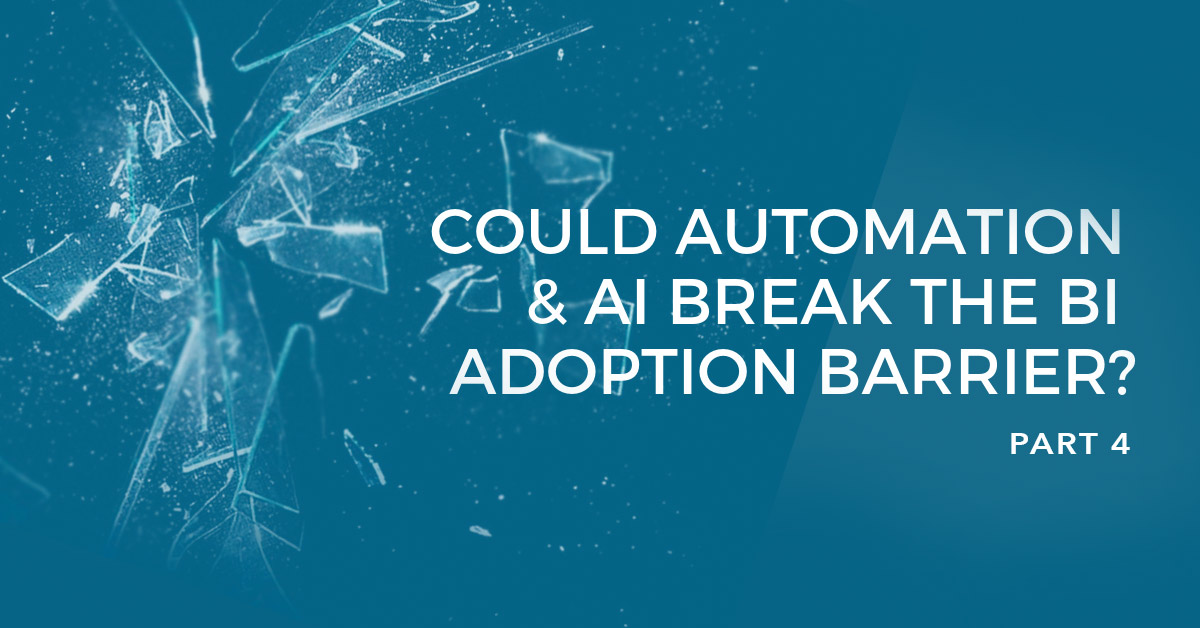
Part 4: How machine learning, AI and automation could break the BI adoption barrier
In the last three parts of this four-part series, we have looked at: research on the state of analytics today and the lack of BI adoption; the history of BI and how we have arrived at the augmented era; and the four main blockers to BI adoption that is stunting the growth of data culture. Today, let's take a look at how AI, machine learning (ML) and automation can close the gap.
How AI-driven technology can close the adoption gap
Algorithms can be highly effective at uncovering hidden patterns and insights within huge volumes of data. Specialists have been using algorithms to solve business problems for years, but the recent advances in processing power have made the development and running of these models more accessible. They can even be run on commodity hardware platforms that are readily available to businesses. This has given rise to the new era of ML-driven analytics.
BI vendors are starting to integrate algorithms into their platforms in a highly intuitive way with the promise of making the tools much more effective at identifying hidden insights and bringing these insights to the enterprise. In the same way that complex GPS technology has become a standard feature on phones that we cannot imagine being without, leveraging algorithms are becoming a standard feature in BI tools. Automating the uncovering of insights and that users might not even be aware that it is operating in the background.
Four ways AI & ML can overcome the adoption blockers
In response to the four issues causing low adoption, here are four ways these technologies, can help overcome these obstacles.
1. Natural interaction
By delivering more intuitive ways to interact with the data, leveraging this underlying technology can help the business surface the insights they need. Through search or menu-based interactions, users can quickly query the data in a manner that is familiar. Alternatively, a click-to-answer functionality allows users to click on a data point, such as a spike, and get an explanation as to why that spike and automatically visualizing the data behind it. More natural querying methods like these suddenly make the ability to surface insights more accessible to the wider business.
For the data analyst, the engines can scan data during the preparation stage and automatically provide profiling information to help identify where data may need improvement or cleaning. Instantly inform analysts of values, ranges, and outliers, and even provide suggestions and automate preparation of a data set.
When it come to analysis itself, tools can also automatically do a lot of the heavy lifting in the background by running algorithms, such as linear regression and ranking, determining when data changes and surfacing the most relevant results. These can then be automatically generated and presented in the form of visualizations within the interface. Depending on the sophistication of the products, they may also visualize correlated data with a similar patten and present that information to the user as well. While correlation doesn't provide causation, coincidence is generally worth surfacing.
2. Natural language assistance
Taking the natural interface one step further, analytics products are also including natural language capabilities. Context is crucial to the interpretation of data, so if the tools can generate a few lines of explanation to inform the reader and provide greater context surrounding the data, it can assist in the analytics being interpreted accurately.
This is a truer form of 'self-service analytics'. Combined with point one above, being able to raise a question of the data in a more natural way or automatically surface a change in the data, with a clear explanation, as opposed to drag and drop building of queries and manually finding that nugget of insight.
3. Reducing time to insight for the data analyst
Automation is also bringing a level of freedom to the data analyst. With the machine doing the heavy lifting, the analyst is freed to focus on the higher value tasks like actual analysis, interpreting the data and translating that into action for the business. Reducing the need to spend time producing a new data visualization for every business query because the technology will automate creation and the data discovery tasks are sped up significantly.
4. Getting the value from predictive analytics
With the integration of predictive models into BI platforms, there has been a bridge built between the analyst and the data scientist. Data science models, such as PMML, can be brought into the data preparation and transformation layer and run against production data. Bringing the models into production with corporate data allows businesses to immediately see the value in a real scenario, on their corporate platform. As seen in the chart below, which shows top analytics priorities for the business, bringing new data models into production is a priority.
The additional power of machine learning
Beyond the traditional analytics front end, algorithms can also be applied across the entire data life cycle. It can be used to detect discrepancies in data, identify outliers, and make recommendations for data modelling. It can also automate standardization across multiple data sources for easy data preparation.
Automation is about freeing people from the tedious tasks to do the more creative work. It is enabling businesses to combine the sheer volume power of machines with the human mind. Automation in analytics is making insights accessible by providing an immediate response when a question is raised. And it is enabling a non-technical audience to better interpret data, which will lead to a much broader adoption of analytics within an organization. This is the future we face and it could revolutionize our approach to analytics and cure the chronic lack of adoption.
Challenges remain
Despite the advances of BI and analytics in the new era of automation, challenges remain. Trust in the data remains an issue but for new reasons. Users need to know they can trust what is under the hood - they will not always be able to see the algorithms and methodologies used. Just as there was once skepticism about the cloud, there will be skepticism about these new capabilities within BI tools. Convincing users of the accuracy of the algorithms, and providing visibility to the methods of which the outcomes are generated, will be paramount to ensuring they trust the tools and therefore the data and insights the machine serves.
Relevancy is another key to adoption. Machines are able to churn out vast numbers of 'insights' on huge volumes of data but if that information are not relevant (e.g there is a spike in air conditioner sales in summer), the user will quickly stop using the tool. ML-generated results may look fantastic and explain vast amounts of detail, but if the insights are not relevant to the particular user at that moment in time, it is useless. The tools will need to build in feedback loops (and some already do) that train the algorithms to serve up insights that matter to that user.
In a similar vein, because users do not want to wade through innumerable analyses served up by the machine, there needs to be the flexibility within BI tools to allow users to define parameters. They need to be able to include or exclude the data they want or specify the type of query they are submitting.
How to accelerate adoption in your business
First, take stock of the analytics solution you have and identify the barriers to adoption that your business is facing. Each business is slightly different and you will need to work on different areas first.
Once the blockers have been identified, ask about your BI tool's roadmap. Every BI vendor has, or will soon have, the ML, AI, and NLP technologies within their platform. But every vendor is applying them differently and using them for different functions. Make sure that the platform you are using meets your business' needs. As this technology will fundamentally change how your business interacts with data, now is the best time to take stock You want your analytics toolset to facilitate your whole business in gleaning data insights, not just your analysts.
Find a tool that automates for everyone
If you want to see adoption across the enterprise, you need to choose an analytics tool(s) that caters to both your data analysts and the business users.
Automation for the data analyst
Analysts should be freed up to do the higher value tasks – actual analysis – and automation can help by doing the heavy lifting. Analysts generally approach data discovery with a question and end goal in mind, so the tool they use should provide them with the ability to apply a targeted and even complex query to the data and be served up the relevant insights they were looking for. With the machine sifting through millions of data points at incredible speeds, the analyst is released from the painfully slow hunting to do the valuable delivery and interpretation of the data.
Automation for the business user
Business users often need data insights almost instantly and they should be complete with explanations that help the user to interpret what the action point should be as a result. This is where natural language explanation can assist in the interpretation of that data.
It's not just all about the machine when it comes to natural language, the context can often happen outside the data and therefore need to be user-driven. Integrating collaboration capabilities enables users to add their own commentary and further external insights to those that are machine generated.
Even when leveraging the machine, the analysis parameters should be able to be defined by the user. This would help prevent the tool from throwing every related data point at the user with the expectation that they will sift through all of them to find the one thing that is relevant. The tool's inbuilt feedback loop should then help the technology to improve the relevance of the insights to each user.
Proactive automation for everyone
With the continued advancement of machine learning and AI in the BI space, you should now be looking for proactive, automated insights. With new automated data discovery products like Yellowfin Signals, users no longer need to check unchanging dashboards, but can be instantly alerted changes in their data – trend changes, outliers, step changes etc. – and any correlating data. These aren’t standard alerts. These are automatically generated by the automation technology, based on data changes that fall outside the norms in your historical data. These automated alerts will come with added contextual tools that allow the user to add further context that can be shared among the team and wider enterprise.
AI could be the key to smashing the adoption barrier
In the BI and analytics self-service world of today, a lack of adoption of the BI tools across the enterprise is a stubborn barrier to the growth of businesses. With barely half of those who have access to data actively accessing the data insights, the vast majority of businesses are still operating on a large portion of gut feel. Over the past decade, adoption has continued to plague the industry despite rapid advances in the speed and sophistication of technologies available.
Data is becoming ever more voluminous, is being delivered faster, and comes in staggering variety. All of this increases the complexity of the data and the queries that therefore need to be formed to extract insights. So, in truth, the advancements in analytics technology have barely kept pace with the growth of the data itself. This means that analytics tools, although more accessible, still require specialist skills to be made truly useful.
But we can now begin to see that augmented analysis and automation could be the solution to the adoption issue. When automation is available to everyone in the enterprise and the grunt work of getting insights is lifted, adoption should see an increase as more people benefit from the BI tools and insights without the effort previously required.
Automation will help remove the barriers of difficult interfaces because the insights will be delivered proactively as notifications and with context provided in natural language. With these freedoms, businesses can expect to see a huge increase in the number of employees interacting with data insights that will inform the everyday business decision making. With data underpinning the decisions, businesses will see an increase in optimization and therefore be able to compete more effectively.
Automation in the BI industry will also dramatically decrease the time to insights as machines can sift through vast volumes of data more accurately and in a fraction of the time that a human can. Data analysts can therefore run more queries and dig deeper into the data answering questions that couldn't be asked before without enormous manpower. And with the integration of data science models directly within the BI platform, businesses will quickly be able to see the value of the predictive data models as they are applied directly to the corporate data.
This technology is about freeing people from the tedious tasks to do the high value work. It is enabling businesses to combine the sheer volume power of machines with the human mind. Automation is making insights accessible and providing a response when a question is raised. And it is enabling a non-technical audience to better interpret data, which will lead to a much broader adoption of analytics within an organization.
Now read the previous blog posts in the series:
Part 1: The state of BI adoption today
Market-leading automation in analytics
Discover Yellowfin Signals - automated data discovery designed to get you to insights in an instant.