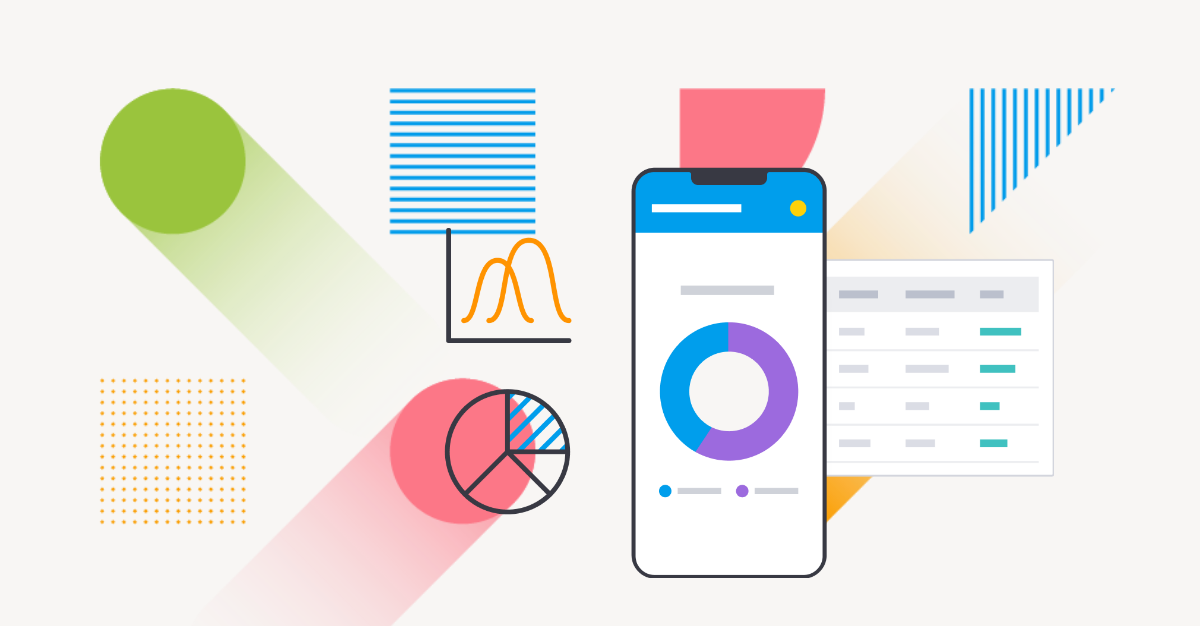
3 Key Drivers and Considerations for AI Analytics in 2025
For independent software vendors (ISVs), creating unique differentiators and value increasingly entails adopting the latest technologies to enhance your product experience, especially in an ever-evolving competitive landscape with multiple new tech solutions.
AI analytics is the latest emerging field in business intelligence (BI) solutions that offers new sophisticated capabilities for the user experience (UX) of your product’s analytics component. But while you may already be familiar with AI analytics, it may be hard to pin-point what factors are driving your competitors towards it, and why you need to prepare.
In this article, we cover the 3 key drivers and considerations for AI analytics today to help provide your business with a better understanding of just how valuable AI-powered analysis is for your users.
Overview of AI analytics
AI analytics refers to the application of artificial intelligence (AI) technologies, including machine learning (ML) and data mining (DM) techniques, to analyze and interpret data.
The term has alternatively been referred to as augmented analytics within the business intelligence industry, and end-users who leverage these tools are named augmented consumers to describe a new class of emerging analytics users who now have access to automated, contextualized, AI-powered capabilities as part of their regular analysis workflow. While these are advanced features, they are also streamlined for non-experts.
Using the approach of AI in analytics enables businesses to extract deeper insights, predict future trends, and automate decision-making processes. AI analytics is distinct from traditional tools as it can handle larger volumes of data and more complex variables.
Recommended reading: What is AI analytics? - Definition & Examples
3 key drivers for AI analytics
When it comes to AI analytics, we have seen three major drivers emerge among our ISV and enterprise customers that have influenced them to accelerate their journey towards implementing AI-powered analytics within their existing products and analytics modules:
Driver #1 - Increased demand for predictive insights
As markets become more competitive, businesses seek predictive capabilities that allow them to anticipate customer needs and market changes. AI analytics provides these predictive insights more accurately and faster than statistical methods, which is a major competitive edge.
By leveraging AI as part of your analytics workflow, you can process vast amounts of data in real-time to forecast market changes, customer demands, and potential risks with a high degree of accuracy. This not only helps in making more informed strategic decisions, but also in optimizing operations and maximizing revenue opportunities. For example, if you are a retail company, you can use AI-driven analytics to predict seasonal buying trends and adjust inventory and marketing strategies accordingly to meet consumer demand without overstocking.
Some AI-powered analytics solutions also often use the technology to streamline the analysis process significantly, meaning that your line-of-business users (i.e. non-data experts or non-analysts) can also take advantage of predictive insights as part of their BI reporting and dashboard exploration without needing specialized training or knowledge.
For example, Yellowfin BI’s Assisted Insights solution allows for detailed, automatically generated analysis or comparisons of datasets with one-click, using natural language to provide an easy-to-understand answer of the ‘why’ behind a particular trend or change that can ultimately help your end-users explore potential forecasts and anomalies without needing to dive into the numbers manually. See it for yourself in the video below.
Learn more: What is Assisted Insights? - How to get to the ‘why’ faster
Driver #2 - Better customer and user experience (UX)
For ISVs, improving the customer experience and UX of your product is one of your most important recurrent objectives. However, refining and personalizing the UX based on feedback can be a long, manual process that may involve a lot of trial and error without the right data to back up proposed changes. This has led more ISVs to seek new capability that helps analyze user behavior and trends faster.
Through machine learning and automated business monitoring, AI analytics enables you to better identify individual customer preferences, buying habits, and engagement patterns, and ultimately deliver more personalized services and products via predictive forecasting and automatic alerting of interesting deviations in your customer datasets. This level of insight allows for the creation of, for example, tailored marketing campaigns and product recommendations, which improve customer satisfaction and loyalty.
For instance, streaming services like Netflix use AI to analyze viewing patterns and provide personalized show recommendations, enhancing user engagement and satisfaction. By continuously learning from customer interactions, AI analytics can help businesses anticipate needs and resolve issues proactively, thereby fostering a positive relationship with customers.
Related reading: How Embedded Analytics Drives Product-Led Growth
Driver #3 - Operational efficiency
AI analytics contributes significantly to operational efficiency by automating routine tasks and optimizing various business processes. By integrating AI into your analytics solutions, you can free up human resources from the time-consuming tasks of data monitoring and preliminary analysis, allowing teams to focus on more complex problem-solving and strategic planning.
Additionally, AI can enhance decision-making processes by providing comprehensive analytics that highlight efficiency bottlenecks and operational gaps. For example, in manufacturing, AI-driven analytics can proactively monitor assembly line data and predict equipment failures before they occur, reducing downtime and maintenance costs.
For a more direct example, Yellowfin BI's Signals feature uses automated business monitoring (ABM) technology (which in itself deploys the use of machine learning and natural language processing) to automatically monitor your data and run analysis to improve efficiency, find interesting statistical outliers and changes, and deliver data alerts and insights to end-users automatically. Watch Signals in action in the video below.
Overall, AI not only streamlines operations but also reduces costs and enhances productivity, making it an indispensable tool for businesses aiming to maintain a lean operational model.
3 key considerations for AI analytics
It’s clear there are many influencing factors behind the rapid rise of AI analytics among ISVs and enterprise organizations today. But before your business can realize any of the value or benefits of this field of technology, you need to address 3 business-critical considerations to prepare for the successful integration and deployment of AI analytics in your product experience.
Consideration #1 - Data governance and quality
Before leveraging AI analytics in any shape or form, it's crucial to establish robust data governance practices and ensure data quality. This involves setting clear policies and procedures on data collection, storage, handling, and sharing to maintain data integrity and security before you use it for analysis and decision-making.
High-quality data is essential for AI systems as it directly influences the accuracy and reliability of the insights generated. Your business must first train and invest in data governance practices that cleanse and validate data, remove duplicates, and fill missing values to ensure the data you are feeding into your potential AI-powered analytics tools is comprehensive and trustworthy.
Establishing a strong data governance framework also helps in complying with modern data protection regulations, such as the General Data Protection Regulation (GDPR), which is crucial for maintaining customer trust and legal compliance in today’s digital dominant landscape.
Read more: What is Data Governance? Accountability and Quality Control in Analytics
Consideration #2 - Skills and expertise
Implementing AI analytics is a step ahead of the fundamental modules of business intelligence solutions, such as BI reporting, dashboards, and data visualization. Because it involves the use of automation and machine-assisted analysis and other AI technologies, you need a team or partnership that can provide specialized skills or training to ensure you have a guiding hand on the long-term value these tools have for your end-users.
ISVs should focus on building or acquiring expertise in the areas of AI through training programs, workshops, and hiring practices. This up-skilling ensures the team can not only develop but also manage and interpret AI-driven analytics effectively.
For smaller organizations or those just beginning with AI, partnering with external experts, consultants or BI solutions vendors that offer AI analytics as part of their broader platform can be a more viable option to bridge the skills gap while you build up internal capabilities gradually.
Consideration #3 - Cultural adoption
For AI analytics to be effective, ISVs must foster a data-driven culture that values and utilizes data in decision-making processes. This cultural shift often requires changing mindsets, training staff to think analytically, and encouraging the use of insights in daily operations.
Your leadership team must champion these changes by demonstrating trust in data-driven decisions, and investing in technologies that integrate analytics into user-friendly platforms. Encouraging open communication about data discoveries and their impact on business outcomes can also help in building an analytics culture within the organization.
For example, Yellowfin BI's data storytellling module, Yellowfin Stories, allows end-users to create blog-style stories to accompany a dashboard or report and provide additional narrative-led context to the numbers; this feature displays who in the business has read, commented and shared the story, helping provide a visible way to build a data culture.
One way to make the integration of AI-powered analytics tools into your product easier is to make analytics synonymous with your user experience. Rather than a separately accessed module, embedded analytics solutions today, such as Yellowfin, specialize in directly integrating analytics, including Stories and the aforementioned AI-powered features, into your product workflows, helping bring data analysis to the end-user.
Learn more: How Embedded Analytics Creates A Data-Driven Culture
Yellowfin BI’s AI analytics features
Yellowfin BI integrates advanced AI analytics capabilities into its business intelligence suite, making it a robust solution for enterprises and ISVs alike seeking to leverage AI analytics. Here is a brief overview of Yellowfin’s AI-specific capabilities, with links to more detailed resources:
Automated insights: Yellowfin's AI algorithms can automatically detect and communicate significant patterns and anomalies in the data, simplifying complex data analysis tasks with its Signals feature.
Predictive analytics: Leveraging machine learning and natural language, Yellowfin can predict trends and behaviors, and explain or compare the significance or particular data-sets to end-users, empowering more people to access insights and use data for proactive decisions with its Assisted Insights feature.
Accessible AI: Yellowfin makes advanced AI tools accessible to non-experts, reducing the barrier to entry for businesses with limited AI expertise, with its Guided NLQ feature.
By focusing on these aspects, businesses can effectively implement AI analytics to drive decision-making and maintain competitive advantage in their respective markets using Yellowfin BI's comprehensive suite of AI tools, while leveraging a scalable, user-friendly analytics platform that aligns with the evolving needs of modern ISVs and enterprises.
Next steps: Explore Yellowfin AI Analytics
Yellowfin is a feature-rich business intelligence and analytics platform with several AI-powered capabilities. Learn more by speaking to our team today.